Recently, the 31st ACM SIGKDD Conference on Knowledge Discovery and Data Mining (KDD) announced its paper acceptance results. A research achievement by Professor Liang Hong’s group from Wuhan University in the field of financial data science applications, titled Disclosing Actual Controller based on Equity Knowledge Graph Learning, has been accepted by the Applied Data Science (ADS) track of KDD. Qingying Xu, a doctoral student in Data Science at our School, is the first author of the paper, with Professor Liang Hong as the corresponding author. Mingxuan Shen, a master’s student in Secrecy Management, and Baokun Yi, an undergraduate student, are co-authors. Our school is listed as the affiliation.
Identifying the actual controllers of companies aids in analyzing and preventing financial risks while providing insights into corporate governance through the lens of control structure. Shareholders exercise voting rights and gain control through their shares. In practice, besides equity relationships, shareholders can form alliances with other shareholders via familial, affiliate, or concerted action relationships to expand their control. However, existing game theory-based methods for identifying actual controllers overlook these non-equity relationships between shareholders and cannot handle large-scale equity networks formed by complex multi-hop relationships due to their exponentially high computational complexity.
To address this issue, Professor Liang Hong’s group constructed an equity knowledge graph to represent the complex semantics and structural information of equity networks, transforming the task of identifying actual controllers into predicting control relationships on the equity knowledge graph. From a financial theory perspective, shareholders who consistently remain in winning coalitions over the long term can be considered actual controllers. To identify stable winning coalitions, the group proposed an Equity Knowledge Graph Learning method (EKGL).
In EKGL, a multi-relation aggregation module was designed to predict the probability of concerted actions among shareholders by integrating different types of relationships between them. On this basis, a meta-path aggregation module captures the shareholding paths of shareholders, encoding the shareholding structure to measure the proportion of shares held. Finally, to determine the winning stable coalition, a control neural network was designed to simulate the voting process of shareholders. Experiments and case studies using real datasets to construct equity knowledge graphs have verified the effectiveness of EKGL.
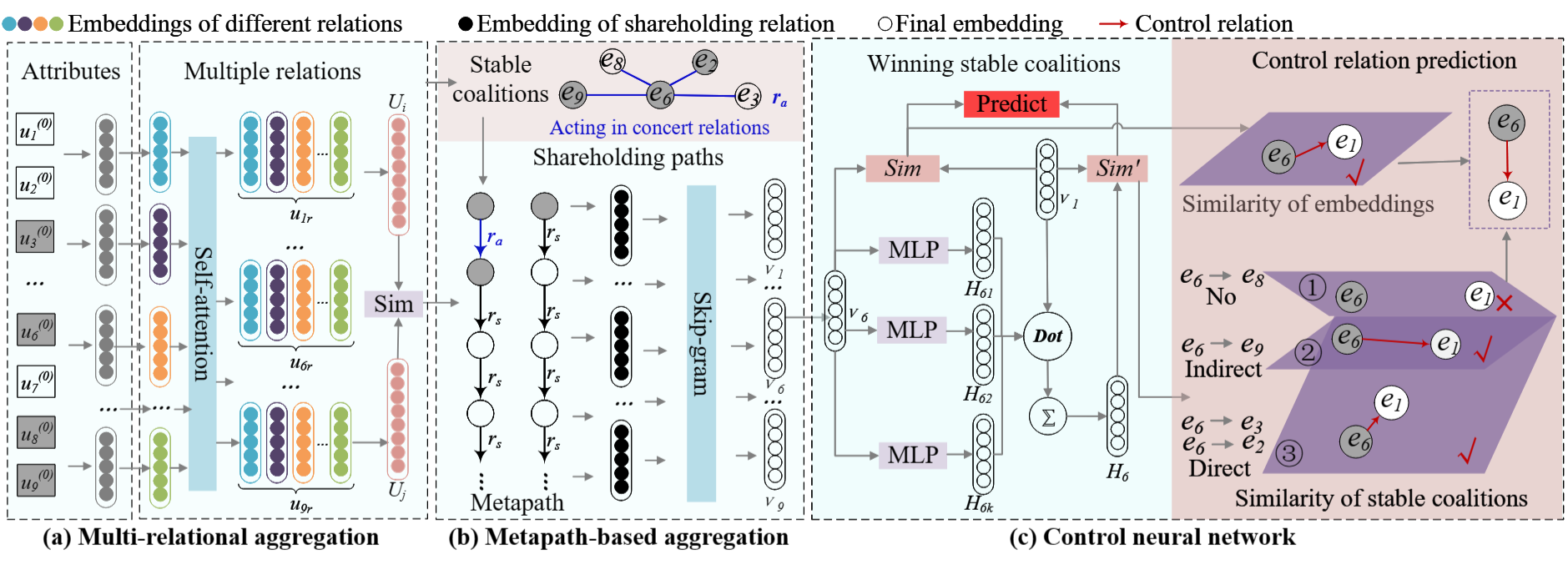
About KDD
KDD is a top-tier international academic conference recommended by the China Computer Federation (CCF) and is one of the premier conferences in the field of data mining. This year, the ADS track received 285 submissions, with only 22% being accepted. This is the first time our school has published a paper in this conference as the corresponding institution.